Eddie Elizondo | Last updated September 2024 | No Hype Analytics blog homepage
As AI technologies and interest continues to evolve rapidly, new terms are constantly being created. Sometimes new terms describe new technologies, and sometimes they are new advertising for the same thing. This is confusing – how do they all connect?
This page is divided into 3 sections:
- A map to cover the main analytics terms from the last 10 years
- Key points from interpreting the map
- An appendix with additional detail for each section in the map
Purists be warned – I am going to take an eye for practical analytics applications with the most simplified yet directionally accurate answer.
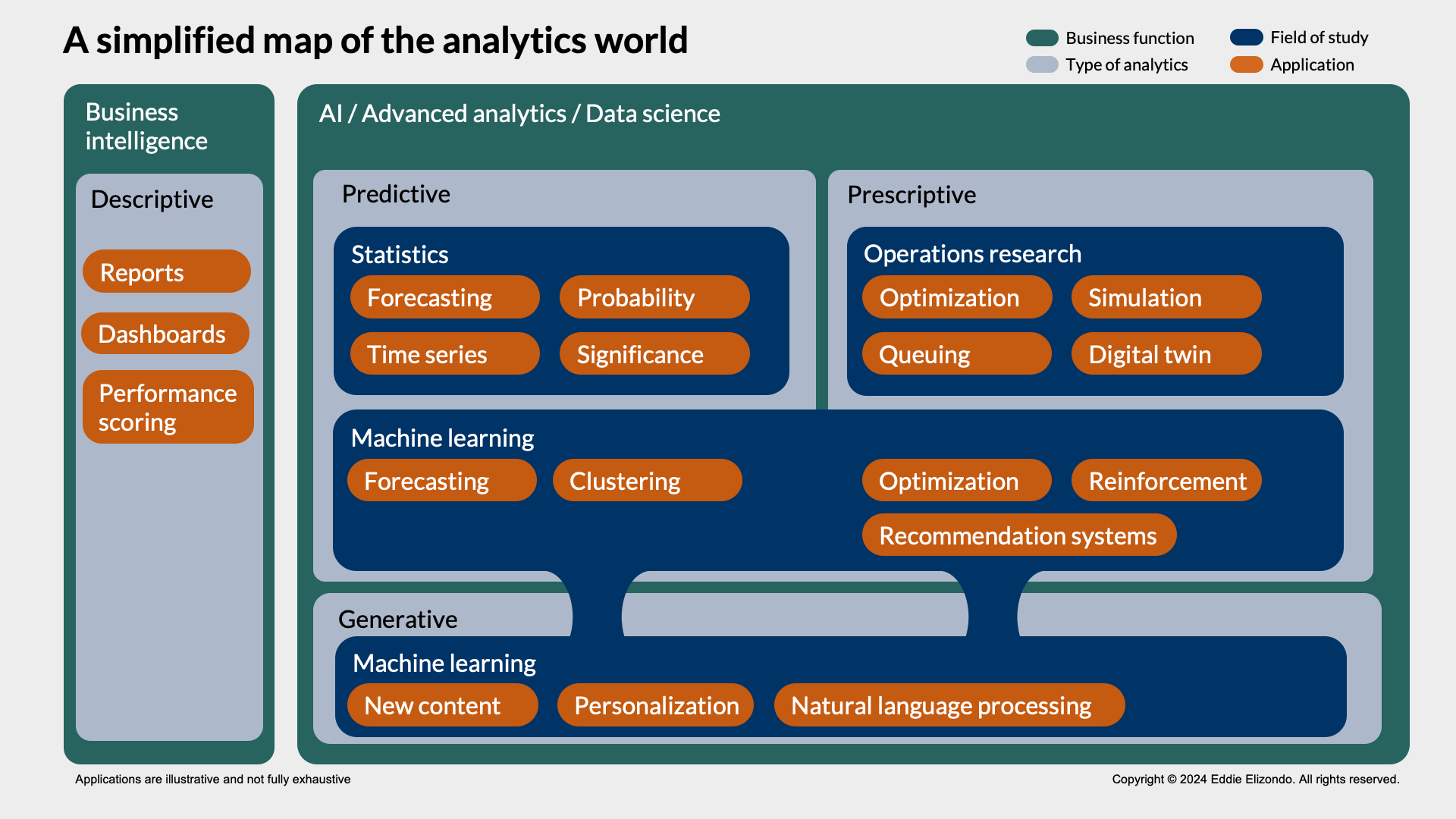
Key points from the map
- Machine learning (ML) has become the most popular and common data science skill because it offers the broadest range of applications. New data scientists get the best bang for their buck learning ML.
- Operations research is a specialized field. It will continue to be a niche part of data science, yet increasingly distinguished and critical for certain applications and industries.
- GenAI was a big deal because it opened a new archetype of analytics.
Appendix – additional detail
Differences between types of analytics
- Descriptive – uses historical data and synthesizes it to better understand the past
- Predictive – applies historical data to probabilistic models explain complex interactions and behavior and forecast the future
- Prescriptive – formulates decisions quantitatively to make optimal choices in the future; used in automation or decision-support systems
- Generative – creates new data as an input for predictive and prescriptive analytics or for standalone use cases
Differences between AI fields of study
- Machine learning (ML): most focused on predicting outcomes or inducing actions by understanding behaviors
- Statistics: high overlap between statistics and ML, with a few exceptions: specialties of statistics are significance testing and experimental design
- Operations research (OR): most focused on managing scarce resources by understanding systems
There is overlap across all three fields, and building AI tools often requires skills across multiple fields. For example, optimizing inventory at a retail store also requires forecasting sales. Generally though, you’ll find:
- OR optimizing factories, logistics networks, pricing of airline seats, and call centers - all of these are optimizing a limited resource (time, goods, employees)
- ML in marketing and forecasting
- Statistics in healthcare and casinos
Since an expert in one field of study is often at least strong in another, how does one know which experts are best fit for a project? Think about what sub-component of the problem will unlock the most value when cracked, and focus expertise there. Also, keep in mind the feasibility of cracking the problem: forecasting is often an initial answer (if only we had a better forecast…), but forecasting remains challenging even with today’s ML tools and has high risk to degrade over time. Like a new car that depreciates when it leaves the sales lot, complex forecasting models can rapidly deteriorate without significant upkeep to maintain performance.
Do you have questions, thoughts, feedback, comments? Please get in touch - I would love to hear from you: eddie@betteroptima.com